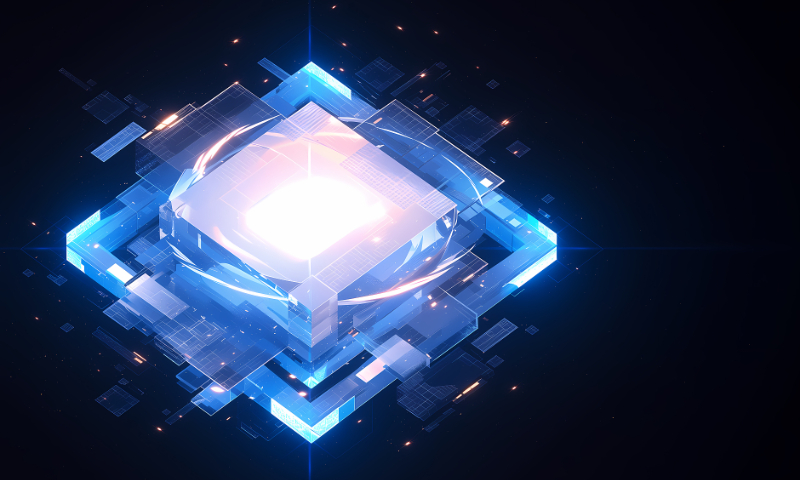
Illustration: VCG
Scientists from Tsinghua University have unveiled a pioneering method for photonic computing that could significantly enhance the training processes of optical neural networks. This advance, coupled with the release of the Taichi-II light-based chip, is poised to offer a more energy-efficient and faster alternative for training large language models.
Chinese researchers Lu Fang and Dai Qionghai, along with their team, published their findings in a paper titled "Fully Forward Mode (FFM) Training for Optical Neural Networks" in Nature magazine. The paper, released on Wednesday, highlights the potential to advance both applied and theoretical fields including deep neural networks, ultrasensitive perception, and topological photonics.
The current standard for training AI models relies heavily on digital computer emulation, which is limited by its high energy demands and dependence on GPU hardware. The FFM learning method developed by Tsinghua allows these computer-intensive training processes to be conducted directly on the physical system, which significantly reduces the constraints of numerical modeling, according to the research team.
While photonic computing has offered high computational power with lower energy consumption compared to conventional methods, it has been restricted to preliminary computations. The precise and complex calculations required for advanced AI training have continued to rely heavily on GPUs, Liu Gang, chief economist at the Chinese Institute of New Generation AI Development Strategies, explained to the Global Times on Thursday.
The new technology developed by Tsinghua's team promises to overcome these limitations, potentially eliminating the need for extensive GPU use and leading to more efficient and precise training of AI models, Liu added.
The first generation Taichi chip, also developed by Tsinghua University and released in April, was featured in Nature magazine. This chip utilizes photonic integrated circuits, which process data using light instead of electrical signals, enabling ultra-fast data transmission and significantly reducing energy consumption.
Compared to its predecessor, the Taichi-II chip has been specifically engineered to perform in-situ training of large-scale neural networks using light, filling a critical gap in photonic computing. This innovation is expected to accelerate AI model training and to excel in areas such as high-performance intelligent imaging and efficient analysis of topological photonic systems.
Energy consumption in the AI industry remains a substantial challenge. According to Rystad Energy, a research institution based in Norway, the combined expansion of traditional and AI data centers, along with chip foundries in the US, is projected to increase energy demand by 177 terawatt-hours (TWh) from 2023 to 2030, reaching a total of 307 TWh. In comparison, the US Energy Information Administration reported that 4,178 TWh of electricity was generated at utility-scale facilities across the US in 2023.
Global Times